
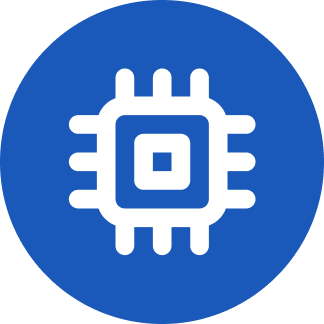
My guess is that it’s more a result of overfitting for alignment. Fine-tuning for “safety” (rather, more corporate-friendly outputs).
That is, by focusing on that specific outcome in training the model, they’ve compromised its ability to give well-“reasoned” “intelligent” sounding answers. A tradeoff between aspects of the model.
It’s something that can happen even in simple statistical models. Say you have a scatter plot of data that loosely follows some trend, and you come up with two equations to describe that trend. One is a simple equation that loosely follows it but makes a good general approximation, and the other is a more complicated equation that very tightly fits the existing data. Then you use those two models to predict future data. But you find that the complicated equation is making predictions way off the mark that no longer fit the trend, and the simple one still has a wide error (how far its prediction is from the actual data) but still more or less accurately fits the general trend. In the more complicated equation, you’ve traded predictive power for explanatory power. It describes the data you originally had but it’s not useful for forecasting data that follows.
That’s an example of overfitting. It can happen in super-advanced statistical models like GPT, too. Training the “equation” (or as it’s been called, spicy autocorrect) to predict outcomes that favor “safety” but losing the model’s power to predict accurate “well-reasoned” outcomes.
If that makes any sense.
I’m not a ML researcher or statistician (I just went through a phase in college), so if this is inaccurate I’m open to corrections.
Well, in some ways. Colorado is already subject to serious drought and water shortage issues; dramatically increased number of/size of/damage from wildfires; temps in excess of 105°f just like, it seems, everywhere else (though CO Springs is higher and cooler than the worst-affected areas of the state, temperature-wise); etc.
Currently in CO Springs it is only 2 degrees (F) cooler than in Huntsville, AL. Humidity is only ~10 pts difference - otherwise yeah I’d say it’s more uncomfortable in AL. Though this is only a snapshot.
I digress.
Point is climate change is affecting (present tense, not future) different areas differently.
You’re absolutely right about the social aspect tho.